Geographically weighted regression helps you to analyze information in a different way. As an alternative of a single, world mannequin, it creates localized fashions, becoming relationships higher inside particular areas. This implies your outcomes aren’t simply averages, they’re extremely particular to the placement, displaying how traits change throughout house. It is like zooming in on totally different elements of a map to know what’s taking place domestically slightly than globally.
This in-depth exploration of geographically weighted regression (GWR) will information you thru its core ideas, methodologies, and functions. We’ll delve into how GWR differs from world regression, emphasizing its capability to seize non-stationary relationships. From understanding information necessities to deciphering outcomes and implementing GWR utilizing varied software program, this complete information offers a whole image of the highly effective spatial modeling approach.
Introduction to Geographically Weighted Regression (GWR)
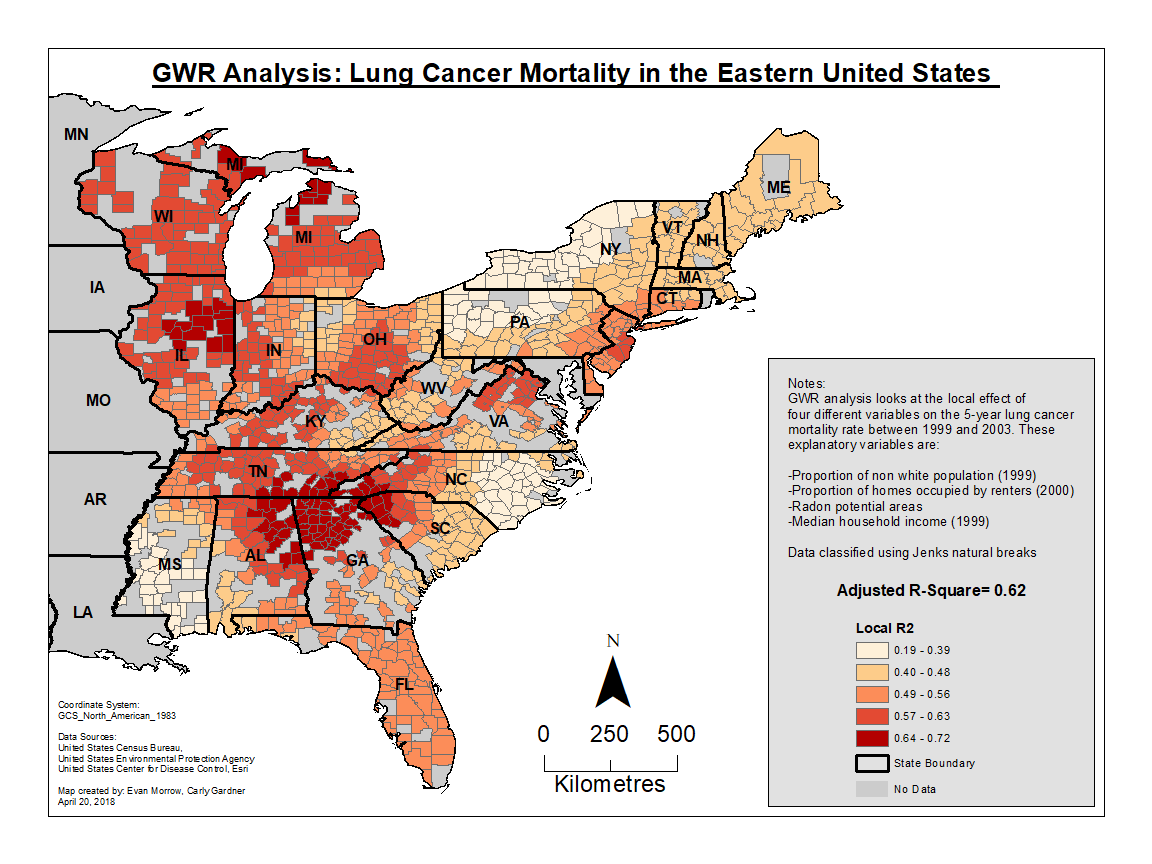
Geographically Weighted Regression (GWR) is a robust statistical approach that acknowledges spatial non-stationarity in information. In contrast to conventional world regression fashions that assume relationships are constant throughout your complete research space, GWR permits relationships to differ throughout totally different areas. This important distinction makes GWR significantly helpful for analyzing phenomena the place native situations considerably affect the end result variable.GWR fashions the connection between variables by estimating native regression coefficients.
These coefficients replicate the distinctive affect of every predictor variable at a particular geographic location. This localized strategy captures spatial patterns and variations that may be missed by a world mannequin, providing a extra nuanced understanding of the underlying processes.
Elementary Rules of GWR
GWR basically differs from world regression fashions by accounting for spatial non-stationarity. International fashions assume a uniform relationship between variables throughout your complete research space. This assumption is usually unrealistic in real-world situations, the place native situations and components can dramatically have an effect on the connection. For instance, the connection between housing costs and earnings may be stronger in city facilities than in rural areas.
GWR addresses this by permitting the connection to differ throughout totally different geographic areas.
Geographically weighted regression helps analyze how issues change throughout totally different areas. For instance, should you have been how reputation of content material like cece_rose onlyfans varies by location, GWR could possibly be a great tool. It is a cool solution to see native traits in information, as a substitute of simply trying on the general image.
Key Assumptions of GWR
GWR, like different regression methods, depends on particular assumptions to make sure dependable outcomes. These assumptions are essential to the validity of the mannequin’s predictions.
- Spatial Dependence: The info ought to exhibit spatial dependence inside particular neighborhoods or areas, which means close by observations usually tend to be related than observations farther aside. This attribute permits GWR to seize the spatial construction of the info.
- No Multicollinearity: Predictor variables shouldn’t be extremely correlated with one another. Excessive multicollinearity can result in unstable coefficient estimates and unreliable mannequin predictions. This implies the unbiased variables do not share an excessive amount of data.
- Usually Distributed Errors: The residuals (variations between noticed and predicted values) must be usually distributed. This assumption ensures that statistical exams utilized in GWR are legitimate.
- Homoscedasticity: The variance of the errors must be fixed throughout all observations. This implies the unfold of the residuals is constant all through the research space.
Comparability with Different Regression Strategies
The next desk compares GWR to different frequent regression methods, highlighting their key variations:
Approach | Description | Spatial Concerns | Assumptions |
---|---|---|---|
Extraordinary Least Squares (OLS) | Estimates a single set of coefficients for your complete dataset. | Assumes a uniform relationship throughout your complete research space. | Assumes fixed coefficients and homoscedasticity. |
Geographically Weighted Regression (GWR) | Estimates coefficients that change throughout totally different geographic areas. | Explicitly accounts for spatial non-stationarity. | Assumes spatial dependence, no multicollinearity, usually distributed errors, and homoscedasticity. |
Core Ideas and Methodology
Geographically Weighted Regression (GWR) is a robust device for analyzing spatial information, providing a extra nuanced perspective in comparison with conventional world regression fashions. It acknowledges that relationships between variables may not be uniform throughout your complete research space. As an alternative of assuming a single set of parameters applies all over the place, GWR permits for the estimation of various parameters for various areas, capturing the native variations within the relationship.
Spatial Dependence
Spatial dependence refers back to the correlation of values between geographically proximate areas. In lots of datasets, values are typically related in areas which are shut to one another. This phenomenon is essential in GWR as a result of it helps to find out the significance of location when estimating relationships. Understanding spatial dependence permits GWR to create native fashions, accounting for the way relationships change throughout the research space.
GWR makes use of spatial weights to emphasise close by information factors within the estimation course of.
Native Regression Parameters
International regression fashions assume a single set of parameters applies all over the place. This may be inaccurate if the relationships between variables change throughout the research space. In distinction, GWR estimates native regression parameters, which means that the coefficients and intercept change from one location to a different. This permits for a extra correct illustration of the native relationships. As an illustration, the impact of promoting expenditure on gross sales may be stronger in densely populated areas than in sparsely populated areas.
Weighting Observations
GWR assigns weights to every statement primarily based on its proximity to the placement being modeled. Observations nearer to the placement obtain increased weights, which means their affect on the native mannequin is bigger. This weighting mechanism displays the concept that close by information factors are extra related in figuring out the connection at a particular location. A standard methodology for assigning weights is utilizing a kernel operate.
Kernel Features
Kernel features are essential for figuring out how weights lower with distance. Completely different kernel features have totally different shapes and properties. The selection of kernel operate impacts the outcomes and must be rigorously thought-about. A Gaussian kernel, for instance, assigns exponentially reducing weights as the space will increase, whereas a uniform kernel offers equal weight to all observations inside a sure distance.
Step-by-Step Process for Implementing GWR
- Outline the research space and variables. Clearly determine the geographical boundaries and the variables you wish to analyze.
- Choose a kernel operate. Select an acceptable kernel operate primarily based on the traits of your information and the anticipated spatial patterns.
- Choose a bandwidth. The bandwidth determines the spatial extent of the affect of observations round a given location. A bandwidth that’s too small might result in overfitting, whereas a bandwidth that’s too giant might end in dropping native variations.
- Estimate the native regression parameters. Utilizing the chosen kernel operate and bandwidth, estimate the parameters of the regression mannequin for every location within the research space.
- Assess the mannequin’s efficiency. Consider the goodness-of-fit of the mannequin for every location, contemplating components reminiscent of R-squared and different related metrics.
- Interpret the outcomes. Study the spatial patterns of the native parameters to know how relationships differ throughout the research space.
Kernel Perform Properties
Kernel Kind | Method (simplified) | Properties |
---|---|---|
Gaussian | exp(-(distance2 / (2 – bandwidth2))) | Clean, quickly reducing weights with distance; appropriate for steady information with easy spatial patterns. |
Biweight | (1 – (distance / bandwidth)2)2, if distance < bandwidth; 0 in any other case | Weights lower quadratically with distance; much less delicate to outliers than Gaussian; appropriate for information with some extent of clustering. |
Uniform | 1, if distance < bandwidth; 0 in any other case | Fixed weight inside the bandwidth; easier however might be much less efficient if relationships are usually not uniform. |
Functions and Use Instances: Geographically Weighted Regression
Geographically Weighted Regression (GWR) is not only a theoretical idea; it is a highly effective device with real-world functions throughout varied disciplines. Its capability to mannequin relationships that change throughout house makes it a worthwhile different to conventional world regression fashions, which assume uniform relationships all over the place. This part explores the varied functions of GWR, highlighting its benefits and showcasing sensible examples.GWR shines when coping with spatial information the place relationships aren’t fixed.
As an illustration, the impact of promoting campaigns on gross sales may differ considerably between city and rural areas, or the impression of air pollution on public well being may differ significantly throughout totally different neighborhoods. GWR permits us to seize these native variations, resulting in extra correct and insightful fashions.
Numerous Functions in Numerous Fields
GWR finds functions in a variety of fields, together with environmental science, city planning, and economics. Its flexibility makes it appropriate for modeling a big selection of phenomena. Take into account its use in predicting air high quality, the place native components like industrial emissions and site visitors patterns are essential, or in city planning, the place the impression of latest developments on property values may differ primarily based on location and proximity to facilities.
- Environmental Science: GWR is beneficial in modeling air pollution dispersion patterns. For instance, scientists can use GWR to mannequin the connection between air air pollution ranges and socioeconomic components (like earnings and training) inside a metropolis. This permits for a extra nuanced understanding of the native impacts of air pollution and might help policymakers tailor interventions to particular areas.
- City Planning: GWR helps in analyzing the impression of city improvement initiatives. Think about a metropolis planning to construct a brand new freeway. Utilizing GWR, they might analyze the impact of the freeway on property values, site visitors patterns, and crime charges in several neighborhoods alongside its route. The evaluation permits planners to see how the results differ from place to position, they usually can thus design extra focused interventions.
- Actual Property Appraisal: GWR can be utilized to foretell property values primarily based on native traits. That is particularly helpful when components like college high quality, proximity to facilities, and neighborhood crime charges have totally different influences in several areas.
Actual-World Situations and Benefits over International Fashions
International regression fashions assume a uniform relationship between variables throughout your complete research space. This assumption might be inaccurate when coping with spatially various relationships. GWR, alternatively, permits for native variations in relationships, resulting in extra correct fashions.
- Instance: Think about predicting home costs in a metropolitan space. A world mannequin may assume {that a} increased crime charge at all times correlates with decrease property values. Nevertheless, GWR can determine areas the place this relationship holds true and areas the place it does not. In sure neighborhoods, different components may be extra influential, like the standard of colleges or entry to transportation.
Geographically weighted regression is cool for determining how issues change throughout totally different areas. It is like zooming in on a map and seeing how issues like crime charges or home costs differ from place to position. For instance, you might use it to investigate how the impression of destiny lane leaks differs in several neighborhoods.
Then, you should use that data to foretell future traits in these areas. Principally, it is a tremendous great tool for understanding patterns in a particular location.
Thus, GWR affords a extra exact image, main to higher predictions.
- Benefit: GWR’s capability to account for spatial heterogeneity is essential when the impact of 1 variable on one other varies considerably throughout totally different elements of the research space. It is a key benefit over world fashions, which fail to seize these variations and supply much less correct predictions.
Spatial Interpolation with GWR
GWR can be utilized for spatial interpolation by predicting the values of a dependent variable at unobserved areas primarily based on the values of predictor variables at noticed areas. That is helpful when information are collected at particular factors, and the values at different areas have to be estimated.
- Instance: Think about mapping the focus of a pollutant in a area. Information may be accessible solely at a couple of monitoring stations. GWR can be utilized to interpolate the pollutant focus at different areas primarily based on components like wind patterns, industrial emissions, and inhabitants density in these areas. This permits for a extra complete understanding of the spatial distribution of the pollutant.
Modeling Non-Stationary Relationships
GWR excels at modeling non-stationary relationships. These relationships change over house. By becoming totally different fashions to totally different elements of the research space, GWR captures these native variations.
- Instance: The impact of promoting on gross sales may be totally different in city and rural areas. In city areas, the impression of a specific advert marketing campaign may be higher as a result of increased inhabitants density. GWR can accommodate these variations, offering a extra correct mannequin than a world mannequin.
Case Examine Desk
Case Examine | Discipline | Description |
---|---|---|
Predicting air high quality in a metropolis | Environmental Science | Modeling the connection between air air pollution and socioeconomic components in several neighborhoods. |
Analyzing the impression of city improvement initiatives | City Planning | Assessing the results of a brand new freeway on property values, site visitors, and crime charges in varied areas alongside its route. |
Predicting property values in a metropolitan space | Actual Property | Modeling the impression of crime charge, college high quality, and facilities on property values, contemplating variations throughout totally different neighborhoods. |
Information Necessities and Concerns
Geographically Weighted Regression (GWR) is a robust device, but it surely’s solely pretty much as good as the info you feed it. Understanding the character of your information, particularly its spatial traits, is essential for profitable GWR evaluation. Several types of information would require totally different dealing with methods, and the presence of lacking values wants cautious consideration. A very good understanding of those components is important to keep away from deceptive outcomes.GWR, in contrast to world regression fashions, permits relationships between variables to differ throughout house.
This makes it important to have spatial information that displays these native variations. In case your spatial information is poorly structured or does not precisely seize the spatial relationships, your GWR mannequin might not be capable to determine significant patterns.
Appropriate Information Sorts for GWR
GWR works greatest with steady information. This contains issues like home costs, earnings ranges, crime charges, or air air pollution ranges. Categorical information can be used, but it surely typically must be transformed into numerical representations (like dummy variables) for the mannequin to course of successfully. The standard of the info, significantly its spatial consistency, considerably impacts the mannequin’s reliability.
Significance of Spatial Information in GWR
Spatial information, together with coordinates and geographic attributes, is key to GWR. It defines the placement of every statement, which is important for calculating the native weights used within the mannequin. Correct spatial information ensures that the mannequin considers the spatial context of every information level. Incorrect or incomplete spatial information can result in inaccurate estimations of native relationships.
For instance, if against the law hotspot is mislocated, the GWR mannequin may not appropriately determine the related components driving the crime.
Challenges in Dealing with Spatial Information for GWR
One main problem is guaranteeing the info’s spatial accuracy. Errors in geographic coordinates or inconsistent projections can result in inaccurate outcomes. One other problem is coping with various spatial resolutions. In case your information factors have totally different ranges of precision, this could have an effect on the mannequin’s capability to seize native patterns precisely. Lastly, coping with sparsely populated areas or areas with only a few information factors can pose challenges.
Native regressions may not be dependable in these areas as a result of restricted pattern dimension.
Coping with Lacking Values in Spatial Information for GWR
Lacking values in spatial information are a typical downside. One strategy is to make use of imputation strategies to fill within the lacking information. Nevertheless, this wants cautious consideration. Imputation methods must be chosen primarily based on the traits of the lacking information. Alternatively, observations with lacking values might be faraway from the evaluation, however this may scale back the pattern dimension and doubtlessly bias the outcomes, particularly if the lacking values are usually not randomly distributed.
It is essential to know the character of lacking values and select essentially the most applicable technique for dealing with them.
Information Codecs Suitable with GWR Software program
Software program | Frequent Information Codecs |
---|---|
R (with packages like `sp` and `gwr`) | Shapefiles, GeoJSON, CSV with spatial coordinates |
ArcGIS | Shapefiles, geodatabases, function lessons |
QGIS | Shapefiles, GeoPackages, CSV with spatial coordinates |
Notice that particular file codecs may differ primarily based on the chosen software program or bundle. It is at all times greatest to seek the advice of the documentation of the particular GWR software program you’re utilizing for essentially the most up-to-date data.
Interpretation and Analysis
Deciphering GWR outcomes goes past merely coefficients. It entails understanding how these coefficients differ throughout house, and the way effectively the mannequin suits the info in several areas. A vital a part of this course of is evaluating the goodness of match, contemplating native significance, and acknowledging spatial autocorrelation’s affect.Understanding the spatial patterns of relationships is vital to deciphering GWR.
As an illustration, a mannequin predicting home costs may present a robust optimistic relationship with earnings in a single neighborhood, however a weaker and even detrimental relationship in one other. This variation displays the native situations influencing the connection between variables.
Deciphering Native Regression Coefficients
Native regression coefficients reveal how a predictor variable’s impact adjustments throughout totally different spatial areas. A coefficient that is optimistic in a single space may be detrimental in one other, suggesting that the predictor’s affect relies on the particular context. Deciphering these native variations is essential for understanding the advanced spatial relationships within the information. For instance, think about a mannequin predicting crime charges.
A optimistic coefficient for inhabitants density in a single space may point out increased crime charges, whereas a detrimental coefficient in one other space may counsel decrease crime charges. It’s because inhabitants density might have totally different results on crime in various environments.
Assessing Goodness of Match
Evaluating the match of a GWR mannequin is much like different regression strategies, however with a spatial twist. Metrics like R-squared, adjusted R-squared, and root imply squared error (RMSE) are essential. Nevertheless, these metrics alone aren’t sufficient. In GWR, the spatial distribution of those metrics is equally essential. A excessive R-squared in a single space may be offset by low R-squared in one other, impacting the general mannequin match.
As an illustration, a excessive R-squared in a rich neighborhood however low R-squared in a much less prosperous space may counsel the mannequin works effectively in some locations however not others.
Evaluating Significance of Native Coefficients
The importance of native coefficients is essential. Normal statistical exams (like t-tests) are used to evaluate whether or not the native coefficient is statistically totally different from zero. This helps decide whether or not a predictor variable’s impact is really current in a given location or just as a result of probability. For instance, in a mannequin predicting retailer gross sales, a statistically vital optimistic coefficient for promoting spending in a single area may counsel a real optimistic impression, whereas a non-significant coefficient in one other area may imply the promoting technique is not as efficient there.
Spatial Autocorrelation
Spatial autocorrelation, the tendency for related values to cluster in house, performs a important function in evaluating GWR fashions. If the residuals (the variations between noticed and predicted values) exhibit excessive spatial autocorrelation, it suggests the mannequin is lacking some spatial patterns or influences. This might result in inaccurate predictions and interpretations. A mannequin predicting property values may present spatial autocorrelation in residuals, indicating the mannequin hasn’t absolutely captured the results of neighboring properties on values.
Abstract of Metrics for GWR Mannequin Analysis
Metric | Description | Interpretation |
---|---|---|
R-squared (native) | Proportion of variance defined domestically. | Larger values point out higher slot in particular areas. |
Adjusted R-squared (native) | Adjusted R-squared worth, contemplating the variety of predictors. | Related interpretation to R-squared, however accounting for mannequin complexity. |
RMSE (native) | Root Imply Squared Error, domestically. | Decrease values point out higher slot in particular areas. |
Native t-statistics | Statistical significance of native coefficients. | Excessive t-values point out vital coefficients, low t-values counsel non-significance. |
Spatial autocorrelation of residuals | Measure of spatial dependence in residuals. | Low values point out no or little spatial autocorrelation in residuals. Excessive values counsel that the mannequin is lacking some spatial patterns. |
Software program and Implementation
Geographically Weighted Regression (GWR) is highly effective, however its implementation relies on the precise software program. Completely different software program packages supply various ranges of ease of use, options, and integration with different instruments. Selecting the best one can considerably impression your evaluation and modeling effectivity.Selecting the best software program for GWR relies on your current ability set, the complexity of your information, and the broader context of your evaluation.
As an illustration, should you’re already snug with R, utilizing it for GWR may be extra environment friendly. You probably have a robust GIS background, ArcGIS may be a pure alternative. This part will Artikel frequent choices and information you thru primary implementations in every.
Frequent Software program Packages for GWR
A number of software program packages excel at implementing GWR. They differ of their syntax, options, and integration capabilities. Frequent decisions embrace R, Python (with libraries like `spgwr`), and ArcGIS.
- R: R is a well-liked statistical computing surroundings extensively used for information evaluation. It has varied packages for spatial evaluation, together with packages particularly designed for GWR. This makes it a flexible and highly effective possibility for researchers accustomed to R’s programming paradigm.
- Python: Python, one other distinguished alternative, affords libraries like `spgwr` that deal with spatial information evaluation. Its object-oriented strategy and in depth libraries make it accessible to a broad vary of customers.
- ArcGIS: ArcGIS, a well-established GIS software program, integrates GWR performance straight into its geoprocessing instruments. That is significantly helpful for customers already working inside the ArcGIS surroundings.
Steps for Implementing GWR in R
This part offers a step-by-step information to implementing GWR in R. This instance assumes you will have your information prepared and have put in the mandatory packages.
- Set up and Load Needed Packages: Use the `set up.packages()` operate to put in the `sp` and `gstat` packages (for spatial information dealing with) and the `spgwr` bundle (for GWR). Load these packages utilizing `library()`.
- Put together Your Information: Guarantee your information is formatted appropriately for GWR. This sometimes entails having a spatial dataset (e.g., coordinates) alongside along with your dependent and unbiased variables.
- Create a Spatial Information Body: Use the `SpatialPointsDataFrame` operate from the `sp` bundle to create a spatial information body out of your information. This permits R to know the spatial context of your observations.
- Carry out GWR: Use the `gwr()` operate from the `spgwr` bundle. This operate requires your dependent and unbiased variables, the spatial coordinates, and the bandwidth. The bandwidth parameter is essential and desires cautious consideration to make sure the mannequin’s accuracy.
- Consider the Mannequin: Assess the mannequin’s efficiency utilizing diagnostic instruments and metrics supplied by the `gwr()` operate, together with the R-squared worth, and look at the native coefficients to know spatial variations. Take into account the bandwidth choice standards rigorously.
Instance R Code Snippet
“`R# Assuming your information is in a dataframe referred to as ‘mydata’# and ‘coordinates’ is a matrix with longitude and latitudelibrary(spgwr)coordinates(mydata) <- ~Longitude + Latitude mydata <- SpatialPointsDataFrame(coords, mydata) gwr_model <- gwr(mydata$dependent_variable ~ mydata$independent_variable, information = mydata, bandwidth = 100, kernel = "bisquare") #Abstract of the mannequin and additional evaluation. abstract(gwr_model) ```
Making a Software program Comparability Desk
A desk outlining software program decisions for GWR, together with their execs and cons, is useful for decision-making.
Software program | Professionals | Cons |
---|---|---|
R | Versatile, in depth statistical capabilities, customizability, giant group help. | Steeper studying curve for customers new to programming. |
Python (with `spgwr`) | Rising group help, accessible syntax, integration with different Python libraries, potential for sooner processing with optimized libraries. | Doubtlessly requires extra setup and set up in comparison with different instruments. |
ArcGIS | Consumer-friendly interface, built-in with different GIS instruments, appropriate for customers accustomed to GIS surroundings. | Restricted customization in comparison with R or Python, potential efficiency limitations for giant datasets. |
Superior Subjects
GWR, whereas highly effective, has some limitations and extensions. This part dives into extra refined methods, together with dealing with a number of spatial variables, incorporating time into the mannequin, exploring potential pitfalls, and evaluating GWR to different spatial regression strategies. Understanding these superior facets helps you to apply GWR successfully in advanced conditions.
GWR with A number of Spatial Variables
Including a number of spatial variables to a GWR mannequin is a typical want. This implies your dependent variable may be influenced by a number of spatial components concurrently. For instance, home costs may rely upon proximity to a number of facilities like parks, colleges, and public transportation, not only one. Correctly modeling these interactions is essential. You will want to make sure the variables are related and never overly correlated.
Fastidiously think about the potential for multicollinearity, which may inflate commonplace errors and weaken the mannequin’s predictive energy. Strategies like variable choice and regularization might help handle this.
GWR in Spatio-Temporal Modeling, Geographically weighted regression
Geographically Weighted Regression (GWR) might be prolonged to include time-varying relationships. That is referred to as spatio-temporal GWR. That is worthwhile for understanding how relationships between variables change over time in a particular geographic space. As an illustration, analyzing crime charges throughout a metropolis over a number of years. The mannequin will mean you can determine how the impression of spatial components (like socioeconomic standing, presence of sure companies, and so on.) on crime charges adjustments over time.
This permits dynamic evaluation and a extra full understanding of evolving phenomena.
Limitations of GWR
GWR, regardless of its strengths, has sure limitations. One key concern is the potential for overfitting, particularly with a restricted dataset or extremely advanced spatial patterns. Selecting the bandwidth parameter appropriately is essential to keep away from this. A bandwidth that is too slender may seize noise within the information, whereas one which’s too broad may miss vital native variations. Moreover, deciphering the outcomes might be difficult, significantly with many spatial variables.
Lastly, the computational burden can enhance considerably with bigger datasets and sophisticated fashions. These facets require cautious consideration within the mannequin constructing course of.
Comparability with Different Superior Spatial Regression Fashions
A number of superior spatial regression fashions exist. Evaluating GWR to different methods, like geographically weighted Poisson regression (GWPR) or geographically weighted quantile regression (GWQR), is important for choosing the right methodology for a particular analysis query. GWPR is beneficial when the dependent variable is rely information (e.g., variety of accidents), and GWQR is designed to mannequin totally different quantiles of the dependent variable (e.g., to know the distribution of home costs).
Desk: Comparability of Superior GWR Strategies
Approach | Description | Strengths | Weaknesses |
---|---|---|---|
GWR | Primary geographically weighted regression | Comparatively easy to implement | Could not seize advanced spatio-temporal relationships |
Spatio-temporal GWR | GWR prolonged to include time-varying relationships | Captures how relationships change over time | Computationally extra intensive |
GWPR | GWR for rely information | Appropriate for rely information, like crime counts | Much less versatile for steady information |
GWQR | GWR for quantiles | Helpful for understanding distribution of dependent variable | Extra advanced to interpret than commonplace GWR |
Conclusive Ideas
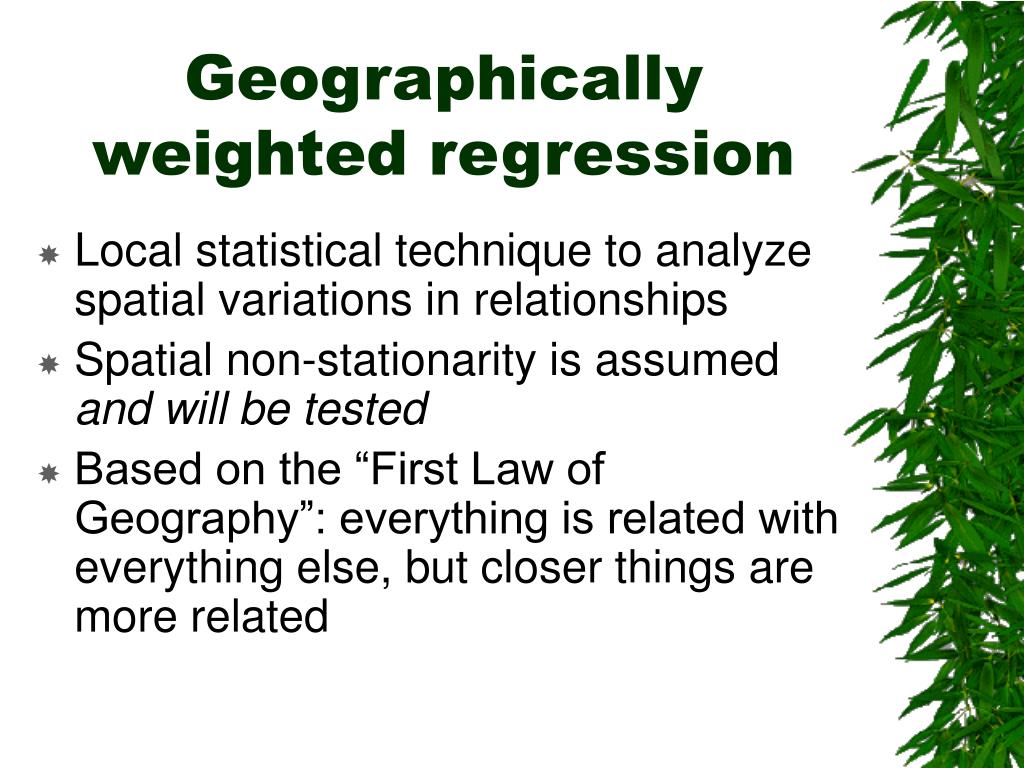
In conclusion, geographically weighted regression affords a versatile and highly effective device for analyzing spatial information. By creating localized fashions, GWR captures advanced relationships higher than world fashions, permitting for a extra nuanced understanding of patterns and traits. This flexibility makes it extremely relevant in various fields like environmental science, city planning, and extra. Armed with this data, now you can deal with spatial information evaluation with confidence and precision, leveraging the strengths of GWR.
Common Inquiries
What are the important thing variations between GWR and Extraordinary Least Squares (OLS)?
OLS assumes a world relationship throughout all information factors, whereas GWR fashions relationships that change domestically. GWR’s energy lies in its capability to seize non-stationary relationships, which means the connection between variables adjustments throughout totally different areas.
How does GWR deal with spatial autocorrelation?
Spatial autocorrelation is a vital side of GWR. The weighting scheme in GWR straight considers the spatial proximity of knowledge factors, implicitly accounting for spatial dependence. The selection of kernel operate additional influences how this spatial dependence is modeled.
What software program can I take advantage of to carry out GWR evaluation?
Well-liked decisions embrace R, Python (with libraries like GeoPandas and statsmodels), and ArcGIS. Every has its personal strengths and weaknesses, and your best option typically relies on the particular information and your familiarity with the software program.
What varieties of information are appropriate for GWR?
GWR thrives on spatial information. You want information factors with related geographic coordinates (latitude and longitude) to seize spatial relationships. Information sorts embrace environmental variables, inhabitants density, and financial indicators.